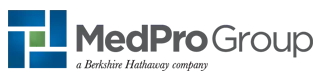
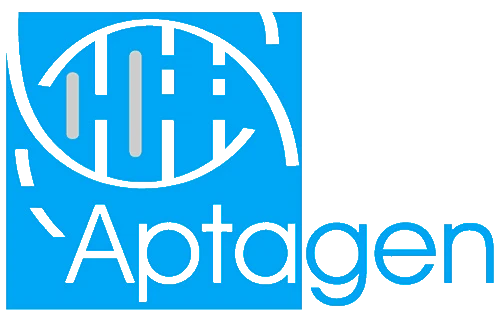
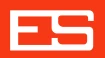

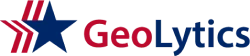

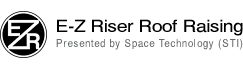
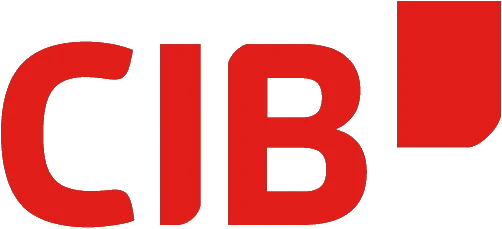
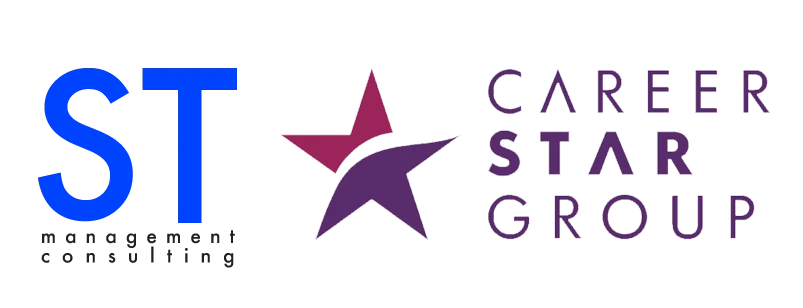
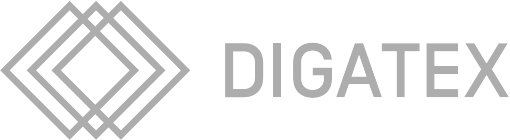
Tailored Solutions For Your Business
Custom Software
Engineering
Azati delivers custom software solutions designed to meet your unique business needs, ensuring seamless functionality, scalability, and optimized performance.
Advanced AI & Machine
Learning
Tailored machine learning models, predictive analytics, and advanced technological implementations by top AI/ML developers to drive innovation in your business.
Data Science
& DWH
We provide expert developers for data-driven solutions – advanced analytics and data management at scale.
DevOps and System
Administration
We offer DevOps engineers who will go your workflows to automate deployments and cloud infrastructure.
Quality
Assurance
The QA experts report early issues, maintain quality performance across various platforms to deliver you with a faultless product.
UX/UI
Design
Azati delivers custom software solutions designed to meet your unique business needs, ensuring seamless functionality, scalability, and optimized performance.
Industries We Cover
Why Choose Us
Fast Project &
Team Setting
Azati often finishes all organizational issues concerning team setting in less than one day and starts project development right away.
Stress-free
Delivery
The development team fulfils customer’s ideas from the most beneficial side and creates the demanded product based on optimal technologies.
Business Goals
Understanding
We are technical experts, focusing on achieving the business goals of our partners and customers through close collaboration with them.
Large Talent
Pool
Azati is providing high-skilled, responsible and proactive specialists and engineers who offer effective solutions and deliver reliable products.
Reasonable
Pricing
Our expertise and reasonable prices have made us a strategic development and consulting partner.
Cooperation
Transparency
We make the development process transparent and help the ideas to evolve in the right direction.
Remote Work
Expertise
20+ years remote work experience helped us to achieve finely honed skills and streamline required business processes for effective workflow.
Outstaffing
Options
Azati is open to providing not only full-staffed teams, but also individual team members to enhance and strengthen the customer’s on-site or external team.